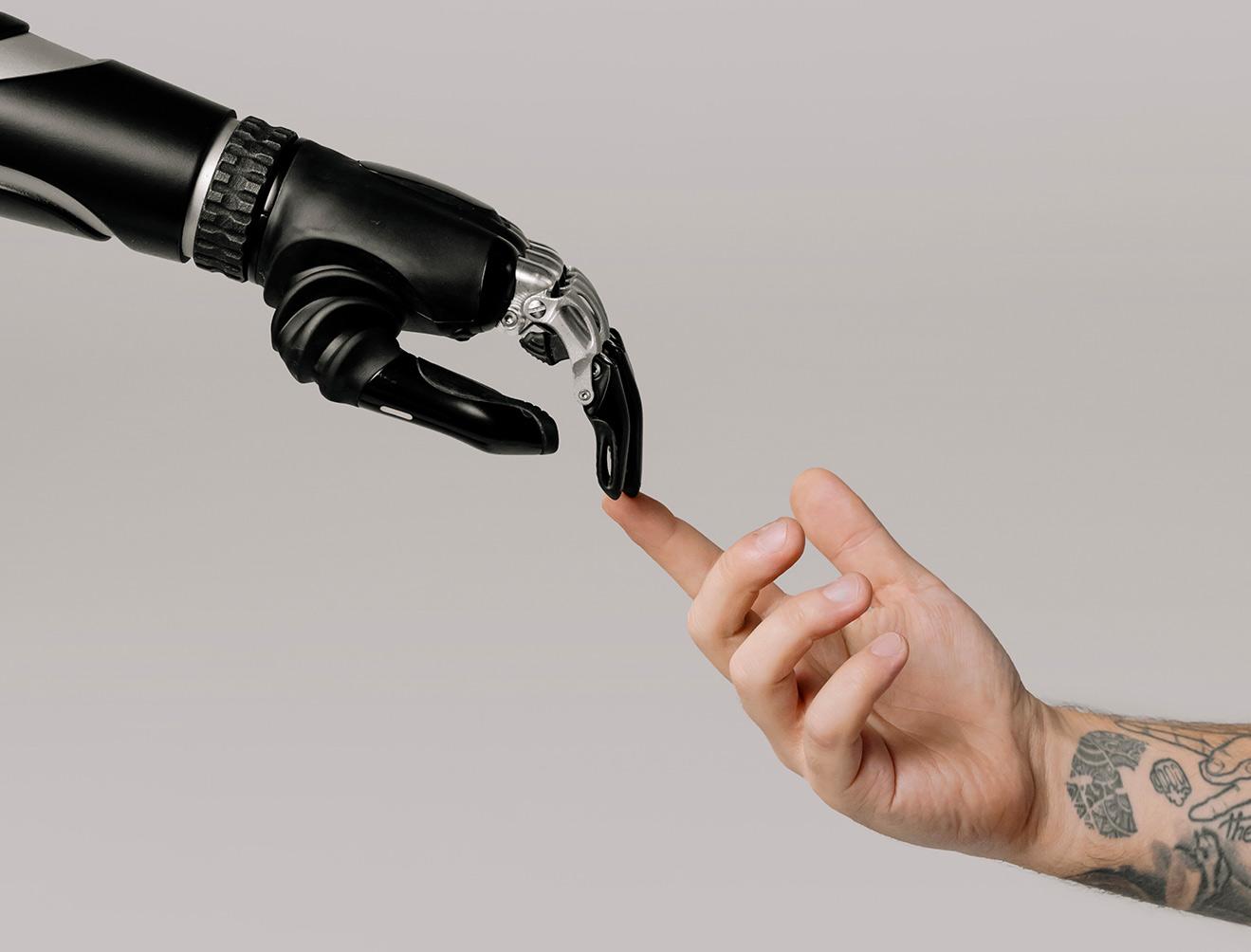
The development of artificial intelligence and other new technologies such as cloud computing and machine learning are already disrupting multiple industries (Artificial Intelligence Disruption, 2015). In the healthcare industry, artificial intelligence (AI) is progressively entering the field of biomedical research and diagnostics. Its utility has been demonstrated in image-based specialities such as radiology, pathology, ophthalmology and dermatology (Yu, Beam and Kohane, 2018). The use of some of these algorithms have received FDA approvals (Scudellari, 2018). In the context of diagnostics and decision-making processes, the widespread adoption of the Electronic Medical Record System (EMRS), at least in developed countries, has enabled the development of AI technologies as soon as in the 1970s, in the form of decision support tools. Yu et al. have highlighted different models regarding the integration of AI approaches in the clinical settings. AI could make not only recommendation based on the information from the EMRS, but could also request data from the EMRS (Yu, Beam and Kohane, 2018). In the extreme scenario, there is the completely automated decision-making process, in which the information provided by the patient would automatically be captured by the AI system, without the intermediary of a clinician or an EMRS. The information gathered by the system would be used to make the diagnosis and the subsequent decision, both would then be integrated in the EMRS.
We have seen the limitation of the information contained in the EMRS, such as the lack of accuracy and the low quality of the data, often uncomplete, unstructured, repetitive or erroneous. These limitations are difficult to overcome. It is hard to ignore in which condition the information was gathered and the clinicians behind the work. To fully benefit from AI approaches, the quality of the data has to be improved.
There is little doubt on the necessity to improve the output of doctors, to improve the accuracy of diagnosis and to reduce the number of medical errors, that could affect up to 5% of the adults (Singh, Meyer and Thomas, 2014). In this context, knowing the discontent of clinicians with the EMRS, but also the needs to improve the quality of medical care, valuable solutions might come from either involving the patients in the process or enhancing the team approach (Singh and Graber, 2015). The challenge lying ahead is how to integrate these recommendations, in a context where EMRS are predominantly consuming most of the time and the attention of doctors. A solution that tackles the following aspects might be helpful: improve the transmission of the information between the patients and the doctors and improve the quality of data in the EMRS.